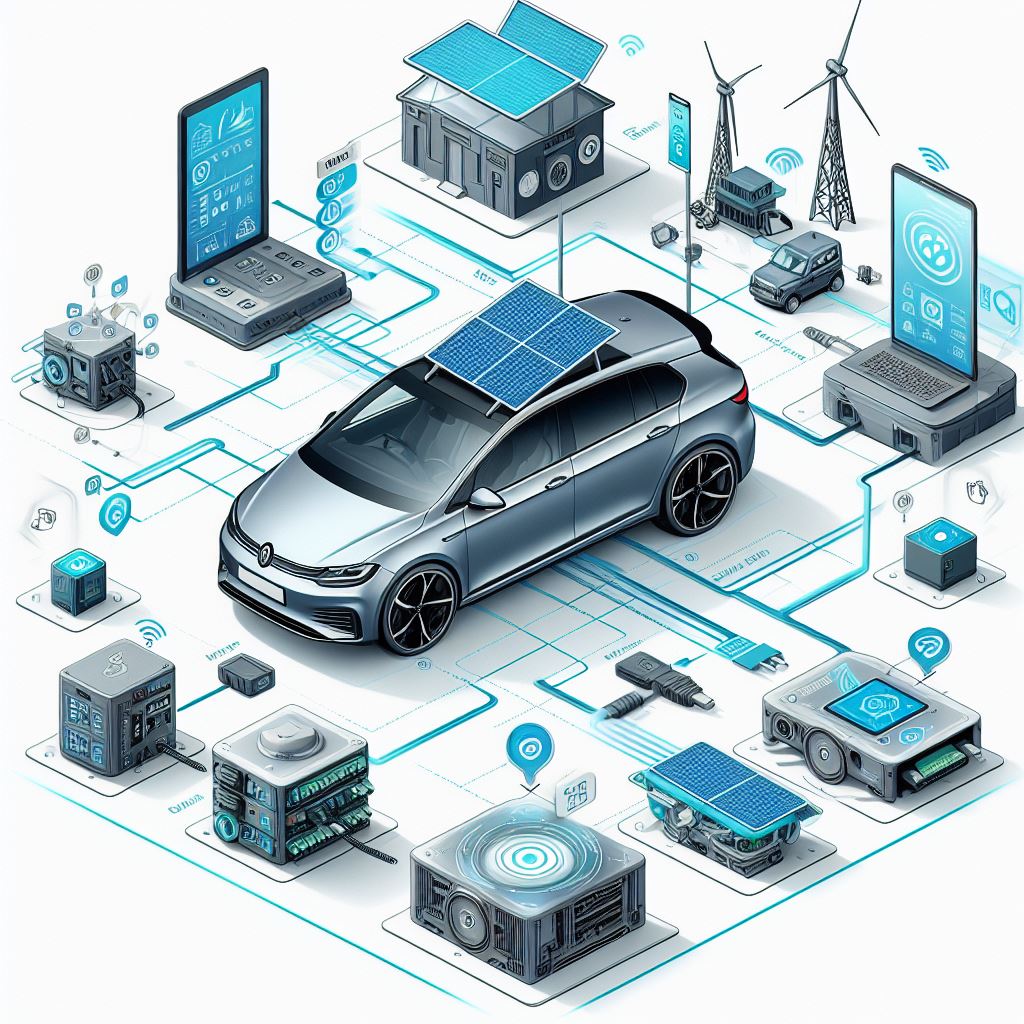
SoftCPS released the PulseCharge prototype.
This IoT system, installed in an Electric Vehicle (EV), enables retrieving real-time data such as the State of Charge (SoC) and Vehicle Identification Number (VIN). It collects this data through command requests sent to an OBD-II adapter, via Bluetooth Low Energy (BLE). PulseCharge then transmits the retrieved data over MQTT/RabbitMQ or any other middleware protocol, to other systems for further processing. The data collected is primarily used for energy applications, which optimize the local energy production with the energy consumption, based on the EnergAIze AI algorithm.
For the system to work, the EV must be equipped with an OBD-II connector. PulseCharge relies on cloud-based systems for storing, analyzing and reasoning on the transmitted information.
PostCharge was developed in the scope of the OPEVA project and the MESCC master, by Ricardo Venâncio. More information, please contact Luis Lino Ferreira.